About

We have released the Waymo Open Dataset publicly to aid the research community in investigating a wide range of interesting aspects of machine perception and autonomous driving technology.
The Waymo Open Dataset is composed of two datasets - the perception dataset with high resolution sensor data and labels for 2,030 segments, and the motion dataset with object trajectories and corresponding 3D maps for 103,354 segments.
What's included
Here's what's currently included in our unique Waymo Open Dataset. We plan to continue growing this dataset, so make sure to continue visiting the page for our most recent updates.
Perception Dataset (last updated March 2024)
[New: March 2024: v. 1.4.3 and v. 2.0.1]
We made small improvements in the 3D semantic segmentation ground truth labels, especially for the class of motorcyclist.2,030 segments of 20s each, collected at 10Hz (390,000 frames) in diverse geographies and conditions
Perception object assets data in a modular format (v2.0.0)
Extracted perception objects from multi-sensor data: all 5 cameras and the top lidar
Lidar features include 3D point cloud sequences that support 3D object shape reconstruction. We additionally provide refined box pose through point cloud shape registration for all objects in the vehicle category
Camera features include sequences of camera patches from the
most_visible_camera
, projected lidar returns on the corresponding camera, per-pixel camera rays information, and auto-labeled 2D panoptic segmentation that supports object NeRF reconstruction, detailed in the paper1.2M object frames from 31K unique object instances that covers 2 classes (vehicles and pedestrians
Check out our related paper, Scalability in Perception for Autonomous Driving: Waymo Open Dataset, for additional details on scale, diversity, and object detection and tracking baselines of the original release.
Sensor data
1 mid-range lidar
4 short-range lidars
5 cameras (front and sides)
Synchronized lidar and camera data
Lidar to camera projections
Sensor calibrations and vehicle poses
Bounding box labels
Labels for 4 object classes - Vehicles, Pedestrians, Cyclists, Signs
High-quality labels for lidar data in 1,200 segments
12.6M 3D bounding box labels with tracking IDs on lidar data
High-quality labels for camera data in 1,000 segments
11.8M 2D bounding box labels with tracking IDs on camera data
2D video panoptic segmentation labels
For a subset of 100k camera images
Labels for 28 classes
Including: Car, Bus, Truck, Other Large Vehicle, Trailer, Ego Vehicle, Motorcycle, Bicycle, Pedestrian, Cyclist, Motorcyclist, Ground Animal, Bird, Pole, Sign, Traffic Light, Construction Cone, Pedestrian Object, Building, Road, Sidewalk, Road Marker, Lane Marker, Vegetation, Sky, Ground, Static, Dynamic
Instance segmentation labels are provided for the Vehicle, Pedestrian and Cyclist classes and are consistent both across cameras and over time
Key point labels
Labels for 2 object classes - Pedestrians and Cyclists
14 key points from nose to ankle
200k object frames with 2D key point labels
10k object frames with 3D key point labels
3D semantic segmentation labels
Segmentation labels for 1,150 segments
Labels for 23 classes
Including: Car, Truck, Bus, Motorcyclist, Bicyclist, Pedestrian, Sign, Traffic Light, Pole, Construction Cone, Bicycle, Motorcycle, Building, Vegetation, Tree Trunk, Curb, Road, Lane Marker, Walkable, Sidewalk, Other Ground, Other Vehicle, Undefined
Map data
3D road graph data for each segment
Includes lane centers, lane boundaries, road boundaries, crosswalks, speed bumps, stop signs, and entrances to driveways
Association of 2D and 3D bounding boxes
Corresponding object IDs are provided for 2 object classes - Pedestrians and Cyclists
Data for 3D Camera-Only Detection Challenge, with 80 segments of 20s camera imagery
Code
Access the GitHub repo here
Check out our related paper, Scalability in Perception for Autonomous Driving: Waymo Open Dataset, for additional details on scale, diversity, and object detection and tracking baselines of the original release
Motion Dataset (last updated March 2024)
Sensor data
1 mid-range lidar
4 short-range lidars
[New]
8 cameras with 360 degree coverage — image embeddings only
[New: March 2024: v 1.2.1 dataset corrections]
The initial release of the WOMD camera data contained misalignment between LiDAR data and roadgraph inputs for some frames. The 1.2.1 release provides new timestamps for the lidar data with an updated pose transformation matrix per time step.
103,354, 20s 10Hz segments (over 20 million frames), mined for interesting interactions
574 hours of data
Sensor data
1 mid-range lidar
4 short-range lidars
Object data
10.8M objects with tracking IDs
Labels for 3 object classes - Vehicles, Pedestrians, Cyclists
3D bounding boxes for each object
Mined for interesting behaviors and scenarios for behavior prediction research, such as unprotected turns, merges, lane changes, and intersections
3D bounding boxes are generated by a model trained on the Perception Dataset and detailed in our paper: Offboard 3D Object Detection from Point Cloud Sequences
Map data
3D map data for each segment
Locations include: San Francisco, Phoenix, Mountain View, Los Angeles, Detroit, and Seattle
Added entrances to driveways (the map already Includes lane centers, lane boundaries, road boundaries, crosswalks, speed bumps and stop signs)
Adjusted some road edge boundary height estimates
Code
Access the GitHub repo here
Check out our related paper, Large Scale Interactive Motion Forecasting for Autonomous Driving: The Waymo Open Motion Dataset, for additional details on the dataset and baseline models.
The Waymo Open Dataset is licensed for non-commercial use. You can find the license agreement here.While this dataset is not reflective of the full capabilities of our systems, and is only a fraction of the data on which Waymo’s autonomous driving system is trained, we believe that for research purposes this large, diverse, and high-quality dataset should be extremely valuable.
Diversity of Geographics
Waymo Open Dataset is collected from a wide variety of places.
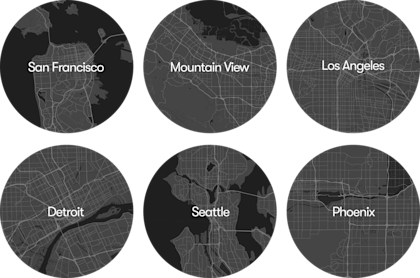
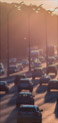
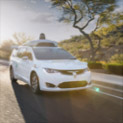
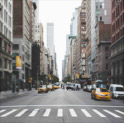
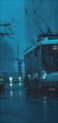
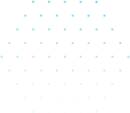
Diversity of Conditions
Waymo Open Dataset includes a wide variety of environments, objects and weather conditions.
Downtown
Suburban
Daylight
Night Time
Pedestrians
Cyclists
Construction
Diverse Weather