August 21, 2019
Waymo Open Dataset: Sharing our self-driving data for research
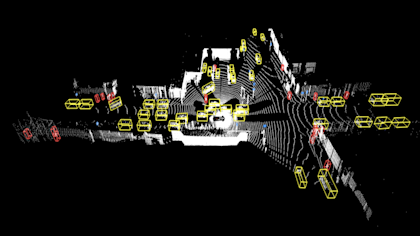
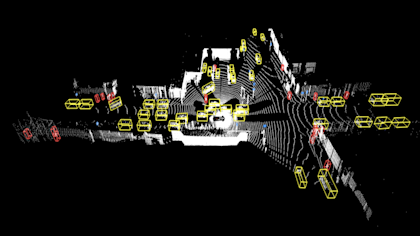
Data is a critical ingredient for machine learning. Our vehicles have collected over 10 million autonomous miles in 25 cities; this rich and diverse set of real world experiences has helped our engineers and researchers develop Waymo’s self-driving technology and innovative models and algorithms.
Today, we are inviting the research community to join us with the release of the Waymo Open Dataset, a high-quality multimodal sensor dataset for autonomous driving.
Available free to researchers at waymo.com/open, it is comprised of high-resolution sensor data collected by Waymo self-driving vehicles. The dataset covers a wide variety of environments, from dense urban centers to suburban landscapes, as well as data collected during day and night, at dawn and dusk, in sunshine and rain.
We believe it is one of the largest, richest, and most diverse self-driving datasets ever released for research.
Size and coverage: This release contains data from 1,000 driving segments. Each segment captures 20 seconds of continuous driving, corresponding to 200,000 frames at 10 Hz per sensor. Such continuous footage gives researchers the opportunity to develop models to track and predict the behavior of other road users.
Diverse driving environments: This dataset covers dense urban and suburban environments across Phoenix, AZ, Kirkland, WA, Mountain View, CA and San Francisco, CA capturing a wide spectrum of driving conditions (day and night, dawn and dusk, sun and rain).
High-resolution, 360° view: Each segment contains sensor data from five high-resolution Waymo lidars and five front-and-side-facing cameras.
Dense labeling: The dataset includes lidar frames and images with vehicles, pedestrians, cyclists, and signage carefully labeled, capturing a total of 12 million 3D labels and 1.2 million 2D labels.
Camera-lidar synchronization: At Waymo, we have been working on 3D perception models that fuse data from multiple cameras and lidar. Waymo designs our entire self-driving system — including hardware and software — to work seamlessly together, which includes choice of sensor placement and high quality temporal synchronization.
When it comes to research in machine learning, having access to data can turn an idea into a real innovation. This data has the potential to help researchers make advances in 2D and 3D perception, and progress on areas such as domain adaptation, scene understanding and behavior prediction. We hope that the research community will generate more exciting directions with our data that will not only help to make self-driving vehicles more capable, but also impact other related fields and applications, such as computer vision and robotics.
This release is just the first step and we welcome community feedback on how to make our dataset even more impactful in future updates.