December 13, 2016
Safely sharing the road with cyclists
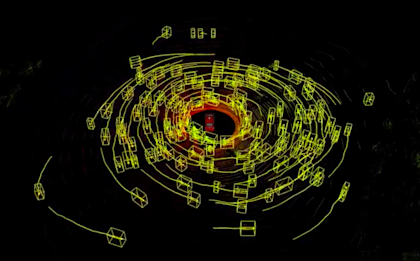
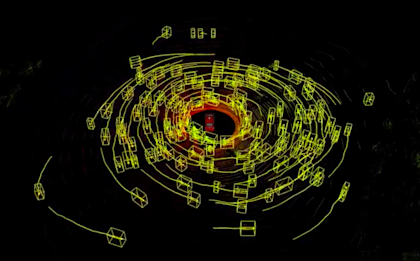
June 2016
Across the U.S., cities have taken steps to keep cyclists safe on the road, whether with separate bike lanes, buffer laws, or share the road campaigns. Still, cyclists are often at risk. In 2014 alone, more than 50,000 cyclists were injured and over 720 were killed on American roads. As cycling becomes more popular (trips more than doubled in the U.S. from 1.7 million in 2001 to 4 million in 2009), it’s important that our self-driving cars share the roads safely with cyclists.
Cyclists are fast and agile — sometimes moving as quickly as cars — but that also means that it’s hard for others to anticipate their movements. Our cars recognize cyclists as unique users of the road, and are taught to drive conservatively around them (it helps to have a number of avid cyclists on our engineering team!).
For example, when our sensors detect a parallel-parked car with an open door near a cyclist, our car is programmed to slow down or nudge over to give the rider enough space to move towards the center of the lane and avoid the door. We also aim to give cyclists ample buffer room when we pass, and our cars won’t squeeze by when cyclists take the center of the lane, even if there’s technically enough space. Whether the road is too narrow or they’re making a turn, we respect this indication that cyclists want to claim their lane.
Through observing cyclists on the roads and private test track, we’ve taught our software to recognize some common riding behaviors, helping our car better predict a cyclist’s course. Our sensors can detect a cyclist’s’ hand signals as an indication of an intention to make a turn or shift over. Cyclists often make hand signals far in advance of a turn, and our software is designed to remember previous signals from a rider so it can better anticipate a rider’s turn down the road.
Because our cars can see 360 degrees, we’re more aware of cyclists on the road — even in the dark. Take, for example, this tricky situation involving two cyclists at night. Our car cautiously approached a cyclist that veered into our lane and stopped to avoid another that suddenly turned a corner and rode directly at us against the flow of traffic. Our car was able to adapt to this unusual situation, and avoid a potential collision.
Bikes can come in many shapes and sizes, so using machine learning we’ve trained our software to recognize many different types. Our software learns from the thousands of variations it has seen — from multicolored frames, big wheels, bikes with car seats, tandem bikes, conference bikes, and unicycles — enabling our car to better share the road no matter your choice of ride.