March 29, 2019
EmTech Digital Recap: How AI Makes Self-Driving Cars Possible
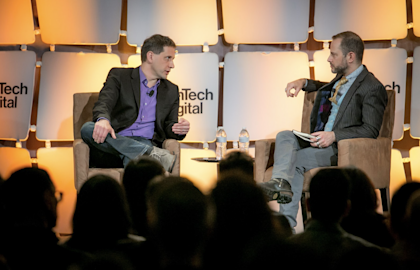
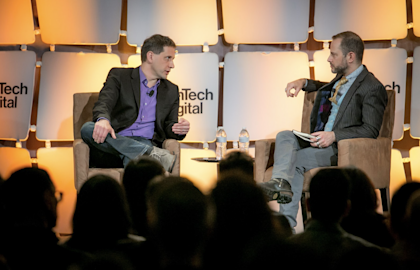
This week our CTO Dmitri Dolgov sat down with MIT Technology Review’s Editor-in-Chief Gideon Lichfield at EmTech Digital. This year’s forum brought together experts from around the world to discuss advancements in AI in a variety of fields, from healthcare and public safety, to transportation and urban design.
Dmitri and Gideon had a wide-ranging conversation on the lessons we’ve learned over the last ten years building the world’s most experienced driver. Below are a few edited highlights:
Q: This year is the tenth anniversary of Waymo’s founding. How far have you come from initial trials and what’s the state of self-driving technology now?
A: A small group of us came together to try and push technology further from early research prototypes to take a step into product and commercialization. Our first order of business was to wrap our heads around what it would take to build a self-driving car. We created two milestones for ourselves: one was to drive 100,000 miles in full autonomy. That was more than anyone had ever done. The second milestone was drive ten routes of 100 miles each in full autonomy from beginning to end. These ten routes were specifically chosen to capture the full complexity of the driving task. We wanted to understand what it meant to drive in suburban areas, freeways and dense urban environments. It took us a little under two years to accomplish both milestones. In the process, we learned a tremendous amount about what it would take to tackle this project.
Q: You’ve been running a self-driving project with cars on the road in Phoenix. Tell us more about that.
A: We kicked off our commercial service] at the end of last year. We took the technology and learned what it means to make a product out of it. It’s an exciting phase for Waymo right now, where we are seeing the effort and investments in technology and experience over the years paying off.
Q: What have been the most interesting AI challenges that have been solved through this process?
A: The whole self-driving industry is very deeply rooted in machine learning and AI. I remember even in the earliest days of the DARPA Grand Challenges, there was some machine learning (ML) that was happening on the Stanford vehicle for terrain classification.
There was, of course, the big transition where deep learning really took off around 2012–2013, and we greatly benefited from those early breakthroughs on our project. At that time, Google was arguably the only company in the world that was investing heavily in both self-driving cars and modern ML. When convolution neural networks and deep learning really took off, we had some researchers work with our colleagues at Google Brain to adapt some of their work on convolution networks to the task that we had of pedestrian classification. It was amazing the amount of performance gained in a very short period of time. The error rate dropped by about a factor of 100.
Since then, we’ve been seeing results in other areas beyond perception, including prediction, understanding intent, understanding how people interact with each other, decision making, and simulation. Nowadays, there’s hardly any part of our system where we don’t use deep learning. As the state-of-the-art in machine learning and AI has evolved, we’ve been adapting our system to use the most advanced algorithms and pushing on the state of the art in many areas on our own.
One thing we’ve learned is that as you bring in new, more advanced algorithms, having a system that already works really well can be to your advantage. You can bootstrap it on the previous system, and it gives you great training data. It gives you a great baseline for comparison, and allows you to iterate much faster.
Q: It seems your focus right now is more on the ride-hailing. You think that’s going to come earlier than personal ownership of cars. Why is that?
A: Ride-hailing is one commercial application we have deployed right now. But our goal is not to build a car. We’re really building a driver.
The core technology — the hardest problems that we’re trying to solve in research and engineering is about building a really good driver. All of the infrastructure, all of the frameworks, and the tools you have to build to evaluate and deploy that driver, are the same. Once you solve the hardest problems, you can put this driver into all kinds of commercial applications, ride-hailing, trucking, deliveries, connecting people to public transit and personally owned vehicles.
Check out the full video here.