August 7, 2019
Why Some Give and Take Could Help Self-Driving Cars Negotiate
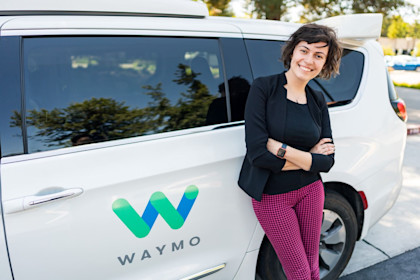
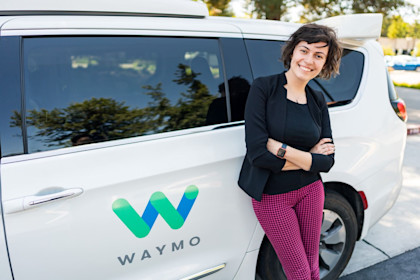
This month Anca Dragan, Waymo research scientist and UC Berkeley assistant professor of electrical engineering and computer sciences (where she also heads up Berkeley’s InterACT lab) was awarded the Presidential Early Career Awardfor Scientists and Engineers. In this post, Anca tells us more about the work that led to the award, and what she is doing at Waymo.
Waymo is looking for talented software and hardware engineers, researchers, and out-of-the-box thinkers to help us tackle real-world problems, advance the state of the art — and make the roads safer for everyone. Come work with other passionate engineers and world-class researchers on novel and difficult problems — learn more at waymo.com/joinus.
Q. How did you find out you won the PECASE award?
I was boarding a plane to Romania, where I was teaching a course, and an email congratulating me came in from someone I recently met at a conference. I thought, “Wait, what?” So, I had to look up my name and “PECASE” in Google and finally found a list of winners on the White House website. My first reaction was to assume it was a mistake because no one had told me, but when I landed, I received an email from the National Science Foundation telling me I’d won and I should come out to DC.
Q. Can you tell us about the research that led to the award?
My project, which was conducted at Berkeley and funded by the National Science Foundation, centered around enabling robots to coordinate with people. More specifically, making robots understand how their actions influence the actions of people around them, and then take that into account during planning. For example, when we share the road, we are coordinating with other road users, and what they do depends on what we do, and vice-versa. The project looked at having robots use this idea of “give and take” so they can have more seamless and efficient interactions while remaining safe.
Q. How does this “give and take” apply to self-driving cars?
First, if you try to predict what everyone else wants to do without accounting for the fact that you can influence them, you end up getting stuck a lot. Imagine trying to merge into traffic, unable to find a large enough gap. Once a self-driving car understands that other people might accelerate or brake when they realize the car is trying to get in, it knows that it could essentially create a big enough gap for itself. In collaboration with students and faculty at Berkeley, our project looked at how to model this mutual influence with game-theory (you can read more about our latest findings in this paper).
Q. What were some of the challenges you encountered?
Besides the computational complexity, one challenge is that there is no universal or single model of human driving. Some drivers are nice, while others are aggressive or inattentive. As part of the project, we modeled driving styles as being optimal with respect to different trade-offs, or priorities, that people have. With that insight, the idea was that we could examine the actions of the other driver to estimate which model best fits.
What we found was that this didn’t work initially, because many drivers behave very similarly if they were just driving forward without any intervention. So what we did was add a small incentive for the robot to gather information and take actions that probe the specific individual’s driving style. The robot would start coming into the lane looking for a response, ready to return if the person didn’t slow down. In another experiment with a 4-way stop, our vehicle would inch forward and see if a person stops to let it go through.
Q: How did you end up working at Waymo?
I was working at Berkeley when I got a call from Waymo. I explained I already had a full-time job, but might be able to consult part-time. I met the folks in the behavior and research teams and knew I wanted to work with them. What’s really special about Waymo for me is that the technology is the state of the art. I could go somewhere else and help them catch up, or I could come here and help drive the most advanced technology forward. As a scientist, the latter seemed much more impactful to me.
Also, I like to work on intricate problems, like the subtleties of how robots coordinate and negotiate with people. To work on that, you need to join a team that has a system that already works well enough. If your system is lacking perception and detecting objects is still your problem, then you can’t really benefit from the work I do because you have a long way to go. It was pretty clear that Waymo was so much further ahead, and that the problems they were facing were a lot of these subtle problems I like tackling.
Q: Was there anything that surprised you when you came to Waymo?
I was really impressed by how knowledgeable people here were on the latest cutting-edge research. And I was also blown away by how much effort has gone not just in developing this technology, but thinking about the challenging intellectual problems that go into testing and validation. As a researcher, I used to think the behavior generation side was the really hard part, but being at Waymo opened my mind to how difficult testing and simulation are as well. Finally, I was impressed by just how much care we give to safety. Around Waymo, you hear “safety is our priority” but when you’re an outsider, you don’t realize how seriously we mean it.
Q: As you get to talk to a lot of people at Waymo, what projects excite you the most?
One project that is really at the cutting edge of research is developing even more realistic agents in simulation. Waymo’s simulator is a powerful tool because we can re-drive miles we have driven before. This is particularly important in giving us quick feedback on changes to our software before they get rolled out to the vehicle. In order for our simulation to be accurate, our simulator needs to account for the fact that the other agents in the scene may change their actions from what happened in real life, too.
Q: What advice would you offer others who are just starting out their career?
My general advice to everyone is to not underestimate the value of a solid mathematical education. The machine learning techniques may change, but the math stays the same. Also, diversify your interests! Don’t stick to one particular field or subfield; try to learn something else and connect the dots to see if things fit together. That’s what is so exciting about dealing with human/robot interactions — I deal with robots, but I’m using a lot of cognitive science and even behavioral economics as well!