September 29, 2022
Benchmarking AV Safety: Demonstrating how the Waymo Driver outperforms the collision responses of always attentive human drivers via industry-leading assessment methods
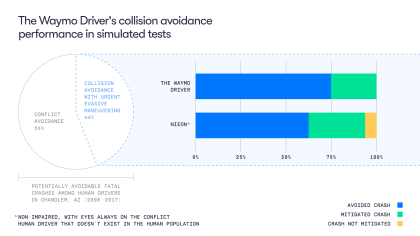
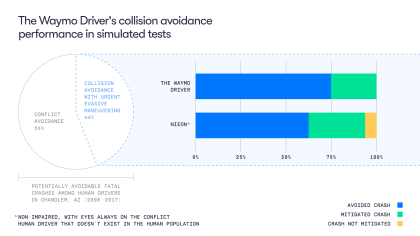
Autonomous driving technology has the potential to dramatically improve road safety and save millions of lives now lost to traffic crashes. Yet, there are still no universally accepted approaches for evaluating the safety of autonomous driving systems. “How safe is safe enough?” and “How do autonomously driven vehicles perform compared to a human driver?” are questions frequently asked across the industry. Following the publication of the Waymo safety framework, real-world performance data, and the simulated reconstruction of fatal crashes, we are taking another important step in answering these complex questions. In our continuous effort to share more information about our safety approaches and metrics, we are releasing two new scientific papers that present methods to compare autonomous vehicle performance to human driving—an important component of determining the readiness of autonomous driving systems.
The Response Time paper presents a framework Waymo uses for analyzing and modeling response timing in a crash-imminent situation on the road. It helps establish how well an attentive and non-impaired human driver avoids collisions to provide a reference point on the spectrum of human driving against which autonomous vehicle performance can be assessed.
Building on that, the Collision Avoidance Benchmarking paper presents a novel methodology to evaluate how well autonomous driving systems avoid crashes. The study, which to our knowledge is the first of its kind, introduces a reference model that represents an ideal human state for driving—the response time and evasive action of a human driver that is non-impaired, with eyes always on the conflict (NIEON). Put simply, unlike an average human driver, NIEON is always attentive and doesn’t get distracted or fatigued¹. The data showed that the Waymo Driver outperformed the NIEON human driver model by avoiding more collisions and mitigating serious injury risk in simulated fatal crash scenarios.
Modeling human driver response time in traffic conflicts
How long does it take a human driver to take evasive action such as braking or swerving to avoid a collision? Until now, human response timing has been mainly evaluated in controlled experiments in an artificial environment, where, for example, subjects are instructed to respond to a well-defined stimulus signal such as a sound or a brake light onset. Inevitably, traditional methods are ill-suited for naturalistic settings like a public road. Road users—such as vehicles, pedestrians, and cyclists—do not always behave based on instructions, and it’s often unclear when to “start the clock”, especially if a traffic situation evolves gradually. Also, traditional methods do not account for the urgency of the scenario. As a result, they tend to overestimate a human driver’s response time in fast-developing situations (often predicting that humans respond later than they actually would), and underestimate it for slower-developing situations (predicting that a human driver would respond earlier than they often do).
Waymo’s proposed framework makes it possible to model human response timing in the real world based on data from naturalistic driving studies. Our model is based on two key ideas. The first is that a human driver’s decision to brake and/or steer to avoid a collision is triggered by a violation to their initial expectations, or in other words, surprise. Thus, response timing always depends on the driver’s current expectations and the onset of surprise defines when to start the clock. The second idea is that human responses depend on the dynamically evolving situation and cannot be represented by a fixed response time applicable to all scenarios. So, if the surprise comes suddenly, for example, when a lead vehicle abruptly brakes hard, the driver will react quickly. Conversely, all other things being equal, a more slowly developing scenario will result in a longer response time.
We used this framework along with an evasive maneuver model to create an internal benchmark for collision avoidance behavior that exceeds that of the typical human driver, which has given us one way to evaluate the Waymo Driver’s performance.
Using a reference behavior model to evaluate the Waymo Driver collision avoidance performance
Last year, we compared the simulated performance of the Waymo Driver to the human drivers involved in a series of fatal crashes that happened between 2008-2017 in Chandler, Arizona. The study showed that the simulated Waymo Driver completely avoided or mitigated 100% of crashes aside from the crashes in which it was struck from behind.
That was a useful measurement because it compared our performance to real drivers on the roads that we operate on in Chandler. While we don’t know the exact circumstances that contributed to those crashes, there are a variety of different factors such as driving while impaired, drowsy, or distracted by secondary tasks that may have been unique to their situation.
To eliminate these factors, we developed a novel model that examines how a non-impaired human with their eyes on the conflict (NIEON)—a consistently performing, always attentive driver that simply does not exist in the human population—would behave in the same exact crashes as we analyzed last year; and compared that to the performance of the Waymo Driver at the time of the study when placed in the responder role in simulated collisions. In other words, we developed a driver model that avoids the human errors that are the most common contributing factors in crashes to provide a higher benchmark of human driving against which to compare the Waymo Driver.
The results are encouraging: the simulated Waymo Driver consistently exceeded that high benchmark. As our previous study showed, the Waymo Driver prevented more than half of potentially avoidable fatal collisions without making any urgent evasive maneuvers at all – for example, by proactively slowing down to let a vehicle in front of us make an unprotected left turn. Our current study extends this prior work by comparing the Waymo Driver and NIEON model in simulations where a conflict was entered. By using evasive maneuvering such as braking or swerving, the NIEON model prevented 62.5% of these crashes and reduced 84% of serious injury risk, but the Waymo Driver was even more effective, as it avoided 75% of these collisions and reduced 93% of serious injury risk.
The goal of this study was to examine a novel method for how a reference behavior model benchmark can be used to evaluate the performance of an autonomous driving system; not to establish exact specifications for it. The following figure provides a conceptual overview of how to interpret the current study’s results in relation to a set of reference points, from the behavior of the drivers involved in the real-world crashes to the complete avoidance of all potential collisions, which is not possible on today’s roadways due to the nature of some multi-vehicle crashes.
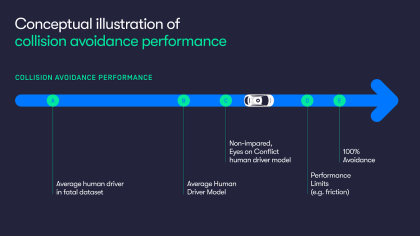
We hope by sharing these methodologies to encourage discussions about safety metrics across the autonomous driving industry, and to provide yet another way to help share and contextualize performance data around autonomous driving technology. We will continue to share more information about our safety approach and encourage other AV companies to join us in this effort. You can learn more about Waymo’s safety framework and previously released performance data at https://waymo.com/safety.
¹In the current study, NIEON was defined by (1) gaze being directed through the windshield toward the forward path during the conflict and (2) a lack of sleepiness and intoxication-related impairment.