June 19, 2021
Waymo at CVPR 2021: Building the future of perception for autonomous vehicles
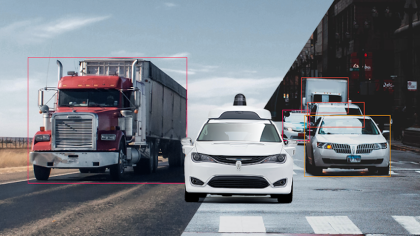
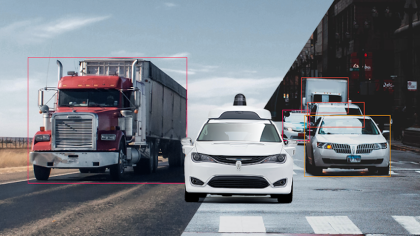
Starting this weekend, researchers, developers, and engineers from all over the world will gather virtually for the 2021 Conference on Computer Vision and Pattern Recognition (CVPR). As a pioneer in the autonomous driving space, Waymo will host and speak in multiple workshops presenting its recent research work.
Chen Wu on Scaling the Perception and Autonomous Driving Systems via Efficient Data, Learning, and Simulation Techniques at ADP3
Charles Qi on Offboard Perception for Autonomous Driving at ADP3
Drago Anguelov on Long Term Prediction in Complex Interactive Environments at The IEEE/CVF CVPR Precognition Workshop
Zoey Yang on The Waymo Open Dataset 2021 Challenges at The Workshop on Autonomous Driving
Pei Sun on Range Sparse Net for Efficient, Accurate Lidar 3D Object Detection
As autonomous driving technology becomes more widespread, researchers will want access to increasing quantities of high-quality data to build robust perception systems, as well as novel architectures that make efficient use of that data. Throughout the next week, the Waymo Research Team will present seven papers that explore building intelligent and flexible computer vision systems.
Some of the models discussed in the papers can identify objects from multiple perspectives and at long distances while others effectively estimate object or scene motion between camera frames and perform a wider range of core computer vision tasks. But one in particular shows the importance of high-quality data from cutting-edge hardware to achieve core tasks in autonomous driving and helps to learn more efficiently from that data.
Offboard 3D object detection: major improvements to the efficient use of data
Models that are both accurate and fast are essential for autonomous driving, when being able to make split-second decisions can be safety-critical. But gathering enough training data to label high-quality precision models can be time-consuming and expensive.
To overcome this, the Offboard 3D Object Detection paper presents new techniques to automatically label the point cloud data from advanced lidar sensors.
Employing the fact that different image frames can capture complementary views of the same object, we’ve designed a novel labeling system that includes multi-frame object detection over time. It also takes advantage of offboard compute to overcome the real-time latency and compute constraints of onboard labeling, ensuring labeling is highly accurate.
The resulting perception pipeline, 3D Auto Labeling, labels data on a par with—if not better than—expert human labelers. That allows rapid increases in the amount of labeled data that can be used to train cutting-edge models—without incurring significant labeling costs or sacrificing label quality when training sparse models.
The Waymo Open Dataset Challenges
This state-of-the-art offboard perception model significantly contributed to the expansion of the Waymo Open Dataset. We used it to annotate the newly released motion dataset -- to our knowledge, the largest interactive dataset yet published for research into behavior prediction and motion forecasting for autonomous driving.
As the Waymo Open Dataset Challenges 2021 have come to an end, a number of teams will present their work at the Workshop on Autonomous Driving at CVPR. This year, we received a lot of strong submissions from participants around the world and were intrigued by the range of creative applications, especially on interesting ideas on core AV perception challenges.
While each submission was unique, we were particularly impressed by the work of Yunnan and David Wu, a father-son team, which, had they been eligible to participate in the challenges, would have ranked first in the Interaction Prediction Challenge leaderboard for their model Air^2. David is a 7th grade student in Palo Alto, who enjoys learning about machine learning and working on real-world problems. Together with his father, David built a robust motion prediction model, while using this opportunity to try out new ideas.
There are so many things behind the scenes other than the model itself that are so important, especially in practical applications,” said David Wu. “I learned how basic machine learning techniques fit into a larger solution, and how a big part of solving a problem is trial and error. All in all, this challenge has taught me so many different things about machine learning, as well as so much practical information, and has granted me such an unforgettable experience. I would like to thank Waymo for organizing such an amazing competition and releasing the Waymo Open Dataset to the public.
Although the contest has ended, the leaderboard remains open for future submissions and we encourage further research and participation. We hope the Waymo Open Dataset will continue to help autonomous vehicle researchers around the world improve their systems and get closer to achieving the true potential of full autonomy.