Waymo Safety Impact
Making roads safer
The trust and safety of the communities where we operate is paramount to us. That’s why we’re voluntarily sharing our safety data.
The data to date indicates the Waymo Driver is already making roads safer in the places where we currently operate. Specifically, the data below demonstrates that the Waymo Driver is better than humans at avoiding crashes that result in injuries, airbag deployments, and police reports.
This hub compares the Waymo Driver’s Rider-Only (RO) crash rates to human crash benchmarks for surface streets. It leverages best practices in safety impact analysis and builds upon dozens of Waymo’s safety publications, providing an unprecedented level of transparency within the autonomous driving industry. By sharing our data and methodologies, we also invite you to join us as we push for advancements in measuring safety impact.
The data displayed on this webpage undergoes consistent updates aligned with NHTSA’s Standing General Order (SGO) reporting timelines.
How the Waymo Driver compares to humans
Rider-only (RO) miles driven
Through December 2024, Waymo has driven 50M rider-only miles without a human driver
The Waymo Driver has tens of millions miles of real-world driving experience. This dashboard shows rider-only miles – miles that Waymo has driven without a human driver — in cities where we operate our ride-hailing service, Waymo One.
Locations |
RO Miles through December 2024 |
---|---|
Los Angeles |
5.165M |
San Francisco |
16.032M |
Phoenix |
28.331M |
Austin |
555K |
Waymo Driver compared to human benchmarks
This table shows how many fewer RO crashes Waymo had (regardless of who was at fault) compared to human drivers with the average benchmark crash rate if they were to drive the same distance in the areas we operate.
The reductions are shown combined and separately for Phoenix and San Francisco. Results have been rounded to the nearest whole number.
The comparisons in Los Angeles and Austin are not shown here due to Waymo’s limited mileage in these cities, which means the results are not yet statistically significant. Comparisons to benchmarks in Los Angeles are available in the download section.
Compared to an average human driver over the same distance in Phoenix and San Francisco, the Waymo Driver had
83% Fewer airbag deployment crashes (65 fewer)
81% Fewer injury-causing crashes (154 fewer)
64% Fewer police-reported crashes (170 fewer)
Phoenix |
33 Fewer airbag deployment crashes |
39 Fewer injury-causing crashes |
77 Fewer police-reported crashes |
San Francisco |
32 Fewer airbag deployment crashes |
115 Fewer injury-causing crashes |
93 Fewer police-reported crashes |
Waymo Driver compared to human benchmarks
Airbag deployments, any injury, police reported
The graphs below show how many fewer incidents (crashes) per million miles (IPMM) Waymo had compared to human drivers with the benchmark crash rate if they would have driven the same distance in the areas we operate. The error bars represent 95% confidence intervals for the IPMM estimate.
The reductions are shown combined and separately for Phoenix and San Francisco.
The comparisons in Los Angeles and Austin are not shown here due to Waymo’s limited mileage in these cities, which means the results are not yet statistically significant. Comparisons to benchmarks in Los Angeles are available in the download section.
Airbag Deployment Crash Rates
Location | Incidents per Million Miles (IPMM), Waymo | Incidents per Million Miles (IPMM), Benchmark |
---|---|---|
Phoenix and San Francisco | 0.29 | 1.76 |
Phoenix | 0.28 | 1.44 |
San Francisco | 0.31 | 2.31 |
Any-Injury-Reported Crash Rates
Location | Incidents per Million Miles (IPMM), Waymo | Incidents per Million Miles (IPMM), Benchmark |
---|---|---|
Phoenix and San Francisco | 0.79 | 4.26 |
Phoenix | 0.74 | 2.12 |
San Francisco | 0.87 | 8.04 |
Police-Reported Crash Rates
Location | Incidents per Million Miles (IPMM), Waymo | Incidents per Million Miles (IPMM), Benchmark |
---|---|---|
Phoenix and San Francisco | 2.19 | 6.02 |
Phoenix | 2.12 | 4.84 |
San Francisco | 2.31 | 8.11 |
Waymo Driver compared to human benchmarks
Percent difference in crash rate
The graphs below show the percent difference between the Waymo and human benchmark crash rates by location, with 95% confidence intervals. A negative number means the Waymo Driver reduced crashes compared to the human driver. Confidence intervals that do not cross 0% mean the percent difference is statistically significant.
The percent reductions and confidence intervals show that the Waymo Driver has a large, statistically significant, reduction in airbag deployment, any-injury-reported, and police-reported crash rate compared to the human benchmark.
The comparisons in Los Angeles and Austin are not shown here due to Waymo’s limited mileage in these cities, which means the results are not yet statistically significant. Comparisons to benchmarks in Los Angeles are available in the download section.
Waymo crash rate percent difference to benchmark
Location | Percent Difference to Benchmark, Airbag Deployment | Percent Difference to Benchmark, Any Injury Reported | Percent Difference to Benchmark, Police Reported |
---|---|---|---|
Phoenix and San Francisco | -83.31% | -81.47% | -63.67% |
Phoenix | -80.39% | -65.03% | -56.21% |
San Francisco | -86.52% | -89.13% | -71.53% |
Percent of Waymo Driver collisions with <1mph change in velocity
(Delta-V <1mph)
Delta-V measures the change in velocity during a collision. It is another way to investigate crash severity and is one of the most important predictors of injury risk in vehicle-to-vehicle crashes.
This graph shows the percentage of SGO-reported crashes where the maximum Delta-V (from either the Waymo vehicle or other vehicle) was less than 1 mph—meaning the collision resulted in a <1mph change in velocity. A Delta-V less than 1 mph usually results in only minor damage (dents and scratches). This graph includes vehicle-to-vehicle and single vehicle crashes, but not crashes with pedestrians, cyclists, and motorcyclists.
Delta-V is estimated using an impulse-momentum crash model with inputs measured by the Waymo vehicle’s sensor system. Note: Comparable human benchmarks for <1mph Delta-V are currently not possible to estimate with high certainty.
% of SGO Collisions with less than 1mph change in velocity (Delta-V <1mph)
Location | % Crashes <1 mph Delta-v |
---|---|
PHOENIX AND SAN FRANCISCO | 47% |
PHOENIX | 43% |
SAN FRANCISCO | 49% |
Waymo Safety Research Partners
David Zuby, Chief Research Officer, Insurance Institute for Highway Safety (IIHS)By making detailed information about crashes and miles driven publicly accessible, Waymo’s transparency will not only support independent research but foster public trust. We hope other companies developing and deploying automated driving systems follow suit.
Methodology
-
Methodology
-
Comparing autonomous vehicle and human performance
Despite the public availability of crash data for both human-driven and autonomous vehicles, drawing meaningful comparisons between the two is challenging. To ensure a fair comparison, there’s a number of factors that should be taken into consideration. Here are some of the most important:
- AV and human data have different definitions of a crash. AV operators like Waymo must report any physical contact that results or allegedly results in any property damage, injury, or fatality, while most human crash data require at least enough damage for the police to file a collision report.
- Not all human crashes are reported. NHTSA estimates that 60% of property damage crashes and 32% of injury crashes aren’t reported to police (Blincoe et al. 2023). In contrast, AV companies report even the most minor crashes in order to demonstrate the trustworthiness of autonomous driving on public roads.
- Focus should be put on injury-causing crashes. Low speed crashes that result in minor damage can cause property damage that can be quickly repaired. These low speed crashes are also the most frequent types of crashes. In traffic safety, the most emphasis is put on reducing the highest severity crashes that can result in injuries.
- It’s important to look at rates of events (incidents per mile) instead of absolute counts. Waymo is growing its operations in the cities we operate in. With more driving miles come more absolute collisions. It’s critical to consider the total miles driven to accurately calculate incident rates. If you do not consider the miles driven, it may appear like incidents are increasing while in reality the rate of incidents could be going down.
- All streets within a city are not equally challenging. Waymo’s operations have expanded over time, and, because Waymo operates as a ride-hailing service, the driving mix largely reflects user demand. The results on this data hub show human benchmarks reported in Scanlon et al. (2023) that are adjusted to account for differences in driving mix using a method described by Chen et al. (2024).
Waymo has used industry best-practices to make a fair comparison between AV and human data sources that is presented on this webpage. This analysis is described more below, and in even more depth in several of Waymo’s safety publications.
-
How we select Waymo incidents noted in this hub
Waymo’s data is derived from crashes reported under NHTSA’s Standing General Order (SGO) and uses the same criteria as described in Kusano et al. (2024).
We are intentionally using publicly available data to allow other researchers to replicate the results. To link the data shown on this dashboard to NHTSA’s published SGO data, researchers can download a list of SGO report IDs and boolean membership in each outcome group in the download section below.
We compare Waymo’s crash rate to human benchmarks across several different types of crashes:
Outcome Description Waymo Data* Human Benchmark Police-reported A crash where a police report is filed Any SGO reported crash with the field “Law Enforcement Investigating” as “Yes” or “Unknown”. Police-reported crashed vehicle rate using state crash data. No underreporting adjustment was applied. Any-injury-reported A crash where any road user is injured as a result of the crash Any SGO reported crash with the field “Highest Injury Severity Alleged” is “Minor”, “Moderate”, or “Serious”, or “Fatality”). “Unknown” reported severity where the SGO narrative mentions injuries of unknown severity are also included. Police-reported crashed vehicle rate where at least one road user had a reported injury. A 32% underreporting adjustment was applied according to Blincoe et al (2023). Airbag deployment A crash where an airbag deploys in any vehicle Any SGO reported crash where the “Any Air Bags Deployed?” is “Yes” for either the subject vehicle (SV) or counter party (CP). Police-reported crashed vehicle rate where any vehicle involved in the crash had an airbag deployment. No underreporting adjustment was applied. *Based on initial data submitted as part of the NHTSA Standing General Order 2021-01
-
Human benchmarks
The human benchmark data are the same as reported in Scanlon et al. (2024). These benchmarks are derived from state police reported crash records and Vehicle Miles Traveled (VMT) data in the areas Waymo currently operates RO services (Phoenix, San Francisco, and Los Angeles). The human benchmarks were made in a way that only the crashes and VMT corresponding to passenger vehicles traveling on the types of roadways Waymo operates on (excluding freeways). The any-injury-reported benchmark also used a 32% underreporting correction (based on NHTSA’s Blincoe et al., 2023 study) to adjust for crashes not reported by humans. The police-reported and airbag deployment human benchmarks rates used the observed crashes without an underreporting correction.
All streets within a city are not equally challenging. If Waymo drives more frequently in more challenging parts of the city that have higher crash rates, it may affect crash rates compared to quieter areas. The benchmarks reported by Scanlon et al. are at a city level, not for specific streets or areas. The human benchmarks shown on this data hub were adjusted using a method described by Chen et al. (2024) that models the effect of spatial distribution on crash risk. The methodology adjusts the city-level benchmarks to account for the unique driving distribution of the Waymo driving. The result of the reweighting method is human benchmarks that are more representative of the areas of the city Waymo drives in the most, which improves data alignment between the Waymo and human crash data. Achieving the best possible data alignment, given the limitations of the available data, are part of the newly published Retrospective Automated Vehicle Evaluation (RAVE) best practices (Scanlon et al., 2024b).
-
Confidence intervals and data limitations
Confidence intervals for Incidents Per Million Miles (IPMM) crash rates were computed using a Poisson Exact method. The confidence intervals for the percent reduction used a Clopper-Pearson binomial described in Nelson (1970). Both confidence intervals were assessed at a 95% confidence level. These confidence intervals use the same methods as described in Kusano et al. (2023).
There is no perfect “apples-to-apples” comparison between human and AV data available today. The benchmarks and comparisons done on this page represent the current state-of-the-art human and AV data sources, based on the state of the art in the research in this field. The airbag deployment benchmark does not have an underreporting correction for the human data because there is no estimate for airbag crash underreporting. Although, it is likely there is more underreporting in human crash data compared to AV crash data. The any-injury-reported benchmark does use an underreporting correction from Blincoe et al. (2023) based on multiple analyses of national crash police-report and insurance data and a national phone survey. It is not straightforward to compute confidence intervals on the any-injury-reported underreporting estimate because it is derived from multiple sources. There is also evidence that underreporting may differ between localities, meaning a national estimate may not fully represent underreporting in the cities Waymo operates in. Similar to the airbag benchmark, the police-reported benchmark has no underreporting estimate even though it is likely there is more underreporting in human data than the AV data (i.e., a crash that meets the reporting threshold is not reported to police).
See Scanlon et al. (2024) and Kusano et al. (2024) for a more comprehensive discussion of the limitations of these results:
- Scanlon, J. M., Kusano, K. D., Fraade-Blanar, L. A., McMurry, T. L., Chen, Y. H., & Victor, T. (2024). Benchmarks for Retrospective Automated Driving System Crash Rate Analysis Using Police-Reported Crash Data. Traffic Injury Prevention (In Press). DOI:10.1080/15389588.2024.2380522.
- Kusano, K. D., Scanlon, J. M., Chen, Y. H., McMurry, T. L., Chen, R., Gode, T., & Victor, T. (2024). Comparison of Waymo Rider-only crash data to human benchmarks at 7.1 million miles. Traffic Injury Prevention (In Press). DOI:10.1080/15389588.2024.2380786.
-
-
FAQ
-
Why is there no local data for Los Angeles and Austin?
Waymo has driven limited miles in certain cities compared to others. When there are limited miles, the comparisons are not statistically significant. We are not showing the results for these areas with limited Waymo miles because the confidence intervals are so large that they would distort the axes of graphs shown on this page. We do present the results from all areas we have driven RO miles in the download section.
-
Why does the hub report on airbag deployment, injury-causing, and police-reported crashes?
Crashes that have airbag deployments, result in injuries, or are reported to police are more relevant to assessing safety than those that result in small amounts of property damage.
-
How often is the data being updated?
In this analysis, we use publicly available data — specifically, Waymo’s crash reports submitted under NHTSA’s Standing General Order (SGO) — to enable other researchers to replicate the results. The data displayed on this webpage undergoes consistent updates aligned with the NHTSA SGO reporting timelines.
In addition to new data being published, we may update the methodology used to do comparisons between the Waymo RO (Rider Only) service and human benchmarks. The best practices in retrospective safety impact is an evolving science. When we do make changes in methodology, we will communicate those changes and their effects on the results and interpretation of the data. For more details, see the release notes documents available in the downloads section.
-
Has your methodology been peer reviewed or validated externally?
This analysis leverages the methodology and human benchmarks introduced in Scanlon et al. (2024) and Kusano et al. (2024).
Both research papers have been accepted for publication in the scientific journal Traffic Injury Prevention, and we anticipate their publication later this year.
Citations:
- Scanlon, J. M., Kusano, K. D., Fraade-Blanar, L. A., McMurry, T. L., Chen, Y. H., & Victor, T. (2024). Benchmarks for Retrospective Automated Driving System Crash Rate Analysis Using Police-Reported Crash Data. Traffic Injury Prevention (In Press). doi:10.1080/15389588.2024.2380522.
- Kusano, K. D., Scanlon, J. M., Chen, Y. H., McMurry, T. L., Chen, R., Gode, T., & Victor, T. (2024). Comparison of Waymo Rider-only crash data to human benchmarks at 7.1 million miles. Traffic Injury Prevention (In Press). doi:10.1080/15389588.2024.2380786.
In addition, the human benchmarks from Scanlon et al. were adjusted using a dynamic driving mix adjustment described by Chen et al. (2024). This paper is currently undergoing the peer review process.
-
Why don’t you share fault information for these collisions?
This analysis included all collisions, regardless of the party at fault and Waymo’s responsibility. Moreover, the question of fault in causing or contributing to a collision is a legal determination. That said, the recent peer reviewed study led by Swiss Re showed that over 3.8 million miles, the Waymo Driver reduced the frequency of property damage insurance claims by 76% and completely eliminated bodily injury claims compared to human drivers.
Citation:
- Di Lillo, L., Gode, T., Zhou, X., Atzei, M., Chen, R., & Victor, T. (2024). Comparative safety performance of autonomous-and human drivers: A real-world case study of the Waymo Driver. Heliyon, 10(14). https://doi.org/10.1016/j.heliyon.2024.e34379
-
Does this data mean the Waymo Driver is safer than humans?
This analysis shows that the Waymo Driver reduces airbag deployment, injury, and police-reportable crashes compared to human drivers in the cities where it operates. Other studies further support these findings and contribute to the growing evidence of the Waymo Driver’s safety benefits. As we accumulate more mileage, it will become possible to make statistically significant conclusions on other subsets of data (for example, LA, Austin, crash types, and more serious types of crashes).
There’s no single metric to evaluate the safety of AVs, and an aggregate, retrospective analysis like this may be one important factor in confirming design elements and predictions done in earlier iterations of our safety determination lifecycle.
-
What about Waymo’s impact on fatalities?
This analysis reviews all crashes that result in any injury – from minor cases to fatal ones – demonstrating that the Waymo Driver outperforms comparable human benchmarks. The Waymo Driver is also inherently designed to mitigate or eliminate the top causes of fatal collisions according to the latest NHTSA data: speeding, impaired and distracted driving, and unbelted passengers.
As we accumulate more mileage, it will become possible to make statistically significant conclusions on other subsets of data (for example, LA, Austin, crash types, and more serious types of crashes).
As has been the case for many safety innovations in the history of vehicle safety, there are other ways to determine the potential of a technology before it is widely deployed and miles are accumulated. For example, our research that reconstructed fatal crashes involving human drivers in Chandler, AZ found the Waymo Driver avoided 100% of simulated, fatal crashes when it was the initiator, and 82% of collisions even when it was the responder. This type of study, when paired with Waymo’s safety readiness determination process, shows that the Waymo Driver has a tremendous potential to reduce serious and fatal injuries.
-
Why does the Waymo SGO data download include crash day, location and zip code?
This information is important to analyze and understand collisions and is not available in the NHTSA SGO.
-
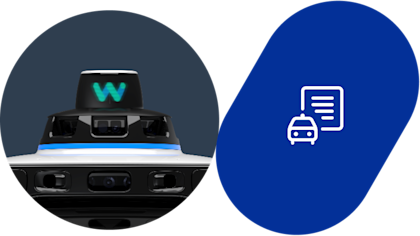
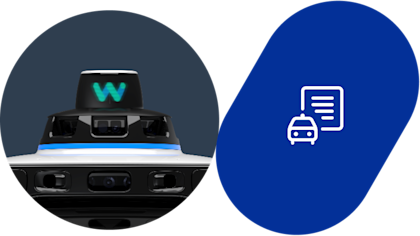
Safety Research
We’re actively conducting studies and publishing peer-reviewed findings on our safety methodologies, performance data, and more
Download Data
-
Miles per Geo
Total miles driven in San Francisco, Phoenix, Los Angeles, and Austin (through December 2024)
Download CSV -
Crashes with SGO identifier and group membership
Police-reported, any-injury-reported, airbag deployment, delta-V < 1 mph and other relevant collision information: day, location, zip code (through December 2024)
Download CSV -
Collision count and comparisons to benchmarks by outcome and location
Aggregated by outcome and location (through December 2024)
Download CSV -
Geographic distribution of benchmark and Waymo RO miles
Human benchmark crash counts for different outcome levels, human vehicle miles traveled (VMT), and Waymo RO miles reported by S2 cell through December 2024. This information can be used to reproduce the dynamic benchmark adjustments.
Download CSV -
Release Notes
A description of changes to the data and methodologies used on the data hub, links to historical data, and data dictionaries.
Download PDF